COVID-19
It has been a few months since December 2019, when a number of people contracted an acute respiratory illness, now known as novel coronavirus-infected pneumonia (NCIP). The outbreak started in Wuhan, Hubei Province, China. As of July, 2020, the disease has rapidly spread around the world, with an estimated 13 million cases. The most common clinical symptoms were fever, loss of taste and smell, cough, weakness and in some cases shortness of breadth. The median incubation period is in average around days. On admission, ground-glass opacity was the most common radiologic finding on chest computed tomography (CT) .
Hematology Parameters
A common step once a patient is admitted to the Emergency Room under the suspicious of COVID is to run a hematology panel along with PCR or antigent tests. The hematology panel consists of two main components: CBC and the DIFF. In the Complete Blood Count (CBC) component, a large number of parameters mainly related to cell concentration and size characteristics of white blood cells, red blood cells and platelets are computed. The measurement of Hemoglobin (Hgb) is also part of the CBC component and this is commonly achieved by shining a light through the solution and measuring how much light is absorbed, the amount of hemoglobin can be determined. The DIFF (Differential) component on the other hand, provides the physician with the distribution of the white blood cells in terms of percentages and concentrations. The figure below shows an example of a common histogram from which counts and percentages are extracted: The WBC histogram. Also, shown below are the five main population of white blood cells. However, a larger number of sub-sets can be defined based on the maturity and morphological changes in the structure of these five basic types.
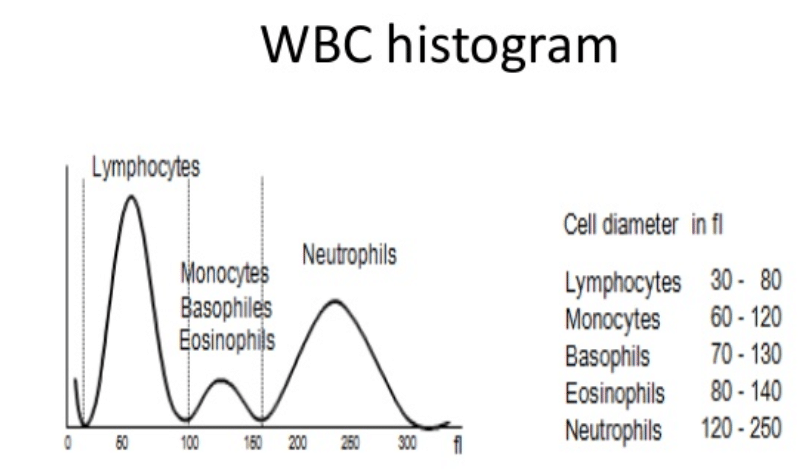
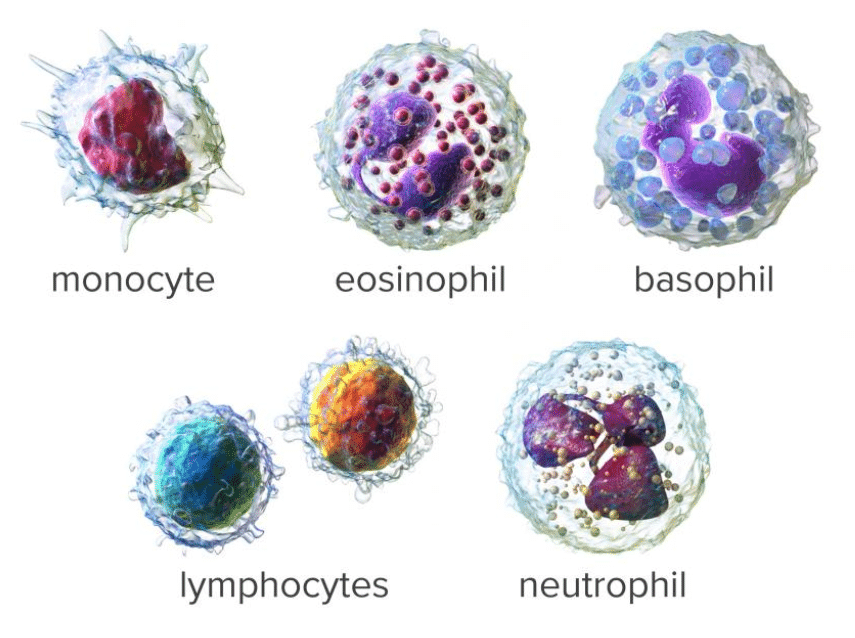
Hematology changes in COVID-19
Recent studies have shown that some of these hematological parameters can show patterns and trends that correlate with the presence and evolution of the COVID infection. In [1] , Lymphopenia was the main affected parameter. Lymphopenia is present when the concentration of Lymphocytes per micro liter of blood drops below 1000 for adults or 3000 for children. Lymphocytes account for 20-40 percent of all white blood cells. Normal Lymphocyte counts for adults are between 1000 and 4800 and for children between 3000 and 9500. In addition, patients who were transferred to the ICU had a deeper nadir (lowest value at a certain point in time) of Monocyte concentration and Hemoglobin. In contrast, they exhibited higher peak Neutrophil concentrations. Neutrophil and Monocytes are white blood cells specialized in killing microorganism such as bacteria. Normal ranges for Monocytes and Neutrophils are in the range of 100-700 and 2500-8000 cells per micro liter of blood.
In [2], Lymphopenia was present in 83.2% of the patients on admission along with Thrombocytopenia in 36.2%, and leukopenia in 33.7% of cases. Patients with severe disease had more prominent laboratory abnormalities than those with non-severe disease. In [3] , a study conducted on a familial cluster of 5 patients with COVID-19 found that all the symptomatic patients had reduced lymphocyte counts. In [4,5], Clinical features of patients infected with 2019 novel coronavirus in Wuhan, China Lymphopenia occurred in 70.3% of patients along with prolonged coagulation times and increased Neutrophil counts. In [6] leucocytes were below the normal range in nine (9%) patients and above the normal range in 24 (24%) patient, Lymphocytes and hemoglobin were below the normal range in many patients.
Affection to the Eosinophil cell population has also been reported in the literature [7,8]. Eosinopenia was associated with the severity of coronavirus disease in patients. The article suggests that early detection of severe COVID-19 based on fever, upper respiratory symptons, lymphopenia and eosinopenia. The pathophysiology for eosinopenia in COVID-19 remains unclear but is likely multifactorial.
A more recent study involving a larger number of patients [10], has shown effects on an ever greater number of hematology parameters and ratios. The study evaluated changes in a large number of parameters, many of which showed significant p-values in the discrimination ability to indentify COVID-19. The long list includes: Lymphocyte count, lymphocyte ratio, platelet count (PLT), white blood cell count (WBC), platelet-large cell ratio, monocyte ratio (MONO%), monocytes count, red blood cell count, red blood cell volume distribution width-CV, red blood cell volume distribution width-SD, hematocrit, mean corpuscular volume, mean corpuscular hemoglobin, mean corpuscular-hemoglobin concentration, mean platelet volume (MPV), basophil ratio (BASO%), basophil count (BASO#), eosinophil ratio (EO%), eosinophil count (EO#), hemoglobin, PLT-I, platelet volume distribution width (PDW), thrombocytocrit, immature granulocytes ratio (IG%), absolute value of immature granulocytes, neutrophil ratio, and neutrophil count.
Dynamic Profile
In [2], hematological and biochemical parameters, were tracked from day 1 to day 19 after the onset of the disease at 2-day intervals. The data collected showed (see Figure below) that non-survivors to the disease developed more severe Lymphopenia over time. White blood cell and Neutrophil counts tended to increase in non-survivors as well.
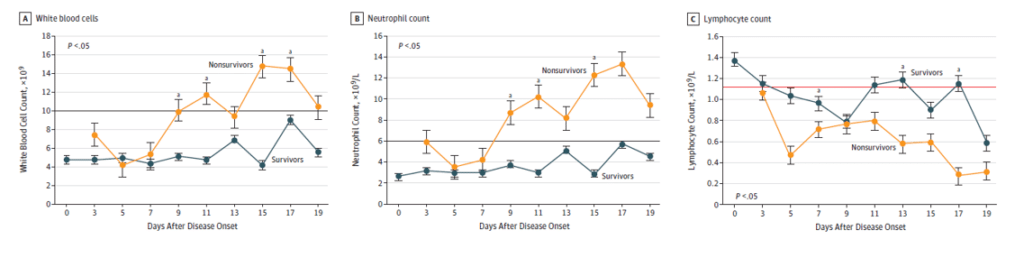
Detection of COVID-19
The gold standard for COVID-19 detection continues to be the RT-PCR (Reverse transcription polymerase chain reaction). It remains the most accurate laboratory method for detecting, tracking and studying the COVID-19 coronavirus. The real time RT–PCR technique is highly sensitive and specific and can deliver a reliable diagnosis in as little as three hours, though laboratories take on average between six and eight hours. Recent advances on deep learning models have drawn attention towards automatic processing of pulmonary images of patients with COVID-19 symptoms. Similarly, in the hematology field, a number of efforts are currently undergoing to build classifiers that receive as input a set of hematological parameters. The classifiers are trained to identified the presence of the disease based on the patterns observed in the labeled training data.
In [6], a number of parameters, combinations and ratios were used to train Fisher’s Linear Discriminant supervised learning model. A linear equation of the Neutrophil to Lymphocyte ration and the red cell size distribution width coefficient of variance yielded an area under the curve (AUC) of 0.923. with a sensitivity of 90% and specificity of 82.4%. Similarly, in [9] an early warning regression model reached an AUC of 0.944 with 93.3% and 72.0% sensitivity and specificity, respectively. In [11], a variety of machine learning models were applied to laboratory tests, including hematology parameters. Decision trees, logistic regression, random forest and support vector machines were developed and evaluated with significant level of success.
It is expected that in the following months, as more hematological data becomes available to the public, a number of automatic classifiers based on machine learning methods will be developed and achieve regulatory approval for the detection of COVID-19. The CBC-DIFF test is relatively cheap, fast and routinely undertaken for most patients. It could add another important data point for the physician decision making process, specially in the emergency room setting.
References
[1] Bingwen Eugene FanVanessa Cui Lian ChongStephrene Seok Wei ChanGek Hsiang LimKian Guan Eric LimGuat Bee TanSharavan Sadasiv MucheliPonnudurai KuperanKiat Hoe Ong Hematologic parameters in patients with COVID-19 infection., American Journal of Hematology. March 2020.
[2] Wei-jie Guan, Ph.D., Zheng-yi Ni, M.D., Yu Hu, M.D., Wen-hua Liang, Ph.D., Chun-quan Ou, Ph.D., Jian-xing He, M.D., Lei Liu, M.D., Hong Shan, M.D., Chun-liang Lei, M.D., David S.C. Hui, M.D., Bin Du, M.D., Lan-juan Li, M.D., et al., Clinical Characteristics of Coronavirus Disease 2019 in China. The new England journal of Medicine, April 2020.
[3] Yan Bai, MD; Lingsheng Yao, MD; Tao Wei, MD; Fei Tian, MD; Dong-Yan Jin, PhD; Lijuan Chen, PhD; Meiyun Wang, MD, PhD. Presumed Asymptomatic Carrier Transmission of COVID-19. JAMA, 2020.
[4]Dawei Wang, MD; Bo Hu, MD; Chang Hu, MD; Fangfang Zhu, MD; Xing Liu, MD; Jing Zhang, MD Clinical Characteristics of 138 Hospitalized Patients With 2019 Novel Coronavirus–Infected Pneumonia in Wuhan, China. JAMA, 2020.
[5] Chaolin Huang, Yeming Wang, Xingwang Li, Lili Ren, Lili Ren, Lili Ren, Clinical features of patients infected with 2019 novel coronavirus in Wuhan, China, The Lancet, February, 2020.
[6] Wang, Changzheng; Deng, Rongrong; Gou, Liyao; Fu, Zhongxiao; Zhang, Xiaomei; Shao, Feng; Wang, Guanzhen; Fu, Weiyang; Xiao, Jianping; Ding, Xiao; Li, Tao; Xiao, Xiulin; Li, Chengbin. Preliminary study to identify severe from moderate cases of COVID-19 using combined hematology parameters. Annals of translational medicine, May 2020.
[7] G. Qian, X. Zhang, A. Ma, N. Yang. Response to: Eosinophil count in severe coronarivus disease 2019., QJM: An International Journal of Medicine, 2020.
[8] Andrew W. Lindsley, , Justin T. Schwartz, , and Marc E. Rothenberg, Eosinophil responses during COVID-19 infections and coronavirus vaccination. J Allergy Clin Immunol. July, 2020.
[9] Xu, Jing; Zhao, Fengde; Han, Mingfeng; Ma, Lei; Zhang, Ting. Zhonghua Wei Zhong Bing Ji Jiu Yi Xue Analysis of the clinical characteristics and early warning model construction of severe/critical coronavirus disease 2019 patients. MEDLINE, April 2020.
[10] H. Yun, Zhuoran Sun, Jun Wu, Aiguo Tang, min Hu, Zhongyuan Xiang., Laboratory data analysis of novel coronavirus (COVID-19) screening in 2510 patients. Clinica Chimica Acta, August, 2020.
[11] Davide Brinati , Andrea Campagner, Davide Ferrari, Massimo Locatelli, Giuseppe Ban, Federico Cabitza. Detection of COVID-19 Infection from Routine Blood Exams with Machine Learning: a Feasibility Study. Journal of Medical Systems. Image & Signal Processing, July 2020.